Improving Continuity of Care among Aging Population
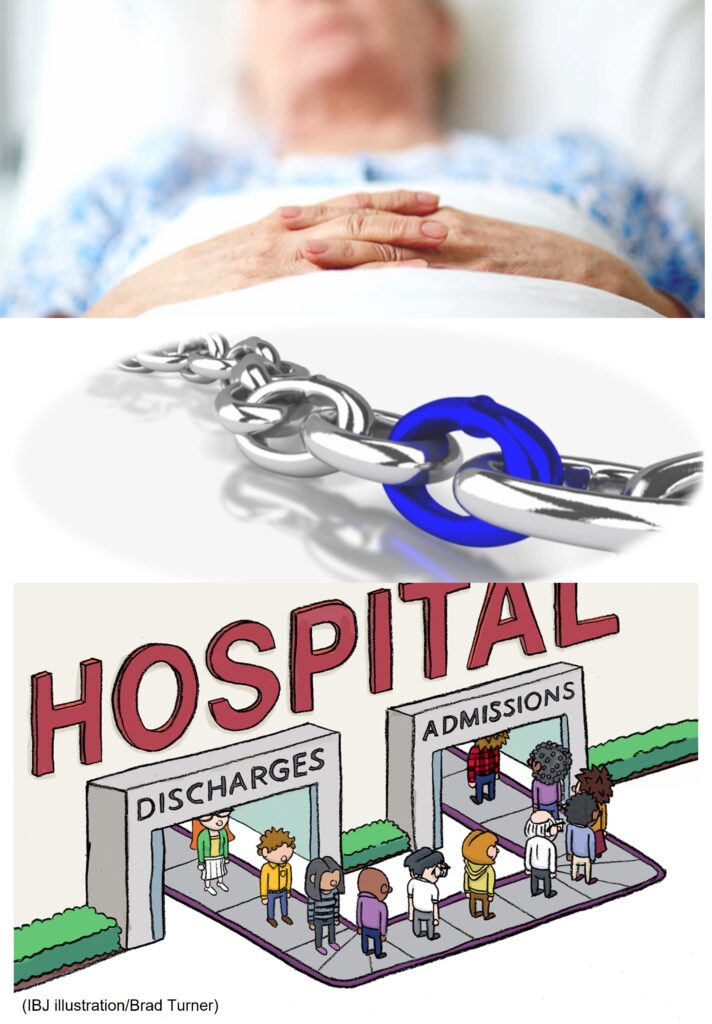
Continuity of care (COC) refers to the delivery of seamless services, continuous caring relationships, and information sharing across care providers. A disruption in COC—that is, care fragmentation (CF)—is an important cause of inefficiency in the Canadian healthcare system; such disruption leads to increased healthcare costs and reduced quality of care. Addressing this issue is particularly challenging among older adults, who often have medically complex needs; such patients can require many care transitions across multiple care settings. An effective strategy for COC improvements is to optimize discharge planning among older adults. However, this is hampered by the imperfect understanding of older patients’ needs, which are associated with their health complexity. Therefore, making early predictions about the patient’s health complexity and incorporating this information into discharge planning decisions can potentially improve COC. I have developed multiple data-driven predictive–prescriptive analytics frameworks that leverage machine learning (ML) approaches and a rich, massive set of longitudinal data collected over a decade. For example, one study investigates the early prediction of older patients’ complexity in hospital pathways using ML. It also examines whether we can conduct accurate prognostics with current information on patient complexity. Another study examines how two common measures of patient complexity—multimorbidity and frailty—concurrently affect post-discharge readmission and mortality among older patients. It also investigates the dependency of the outcomes on other essential socio-demographic factors. The third study examines the feasibility of predicting patients at risk of fragmented readmission—that is, readmission to a different hospital than the initial one. It uses this predictive information to derive optimal policies for preventing CF while addressing disparities in the decision-making process. The findings highlight the feasibility, utility, and performance of predicting patient complexity and important adverse outcomes, potentially undermining COC. These studies show that advanced knowledge and explicit utilization of this information could support decision-making and resource planning toward a targeted allocation at the system level; moreover, it informs actions that affect patient-centered care transition at the service level to optimize patient outcomes and facilitate upstream discharge processes, thereby improving COC.
Sequential Decision Problem for Diabetes Management
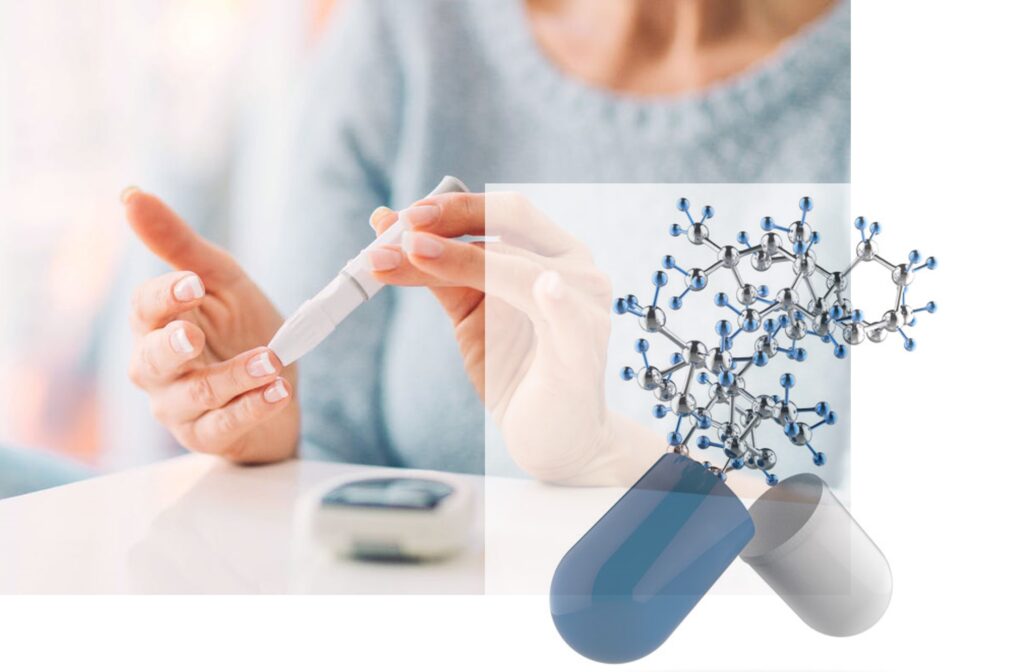
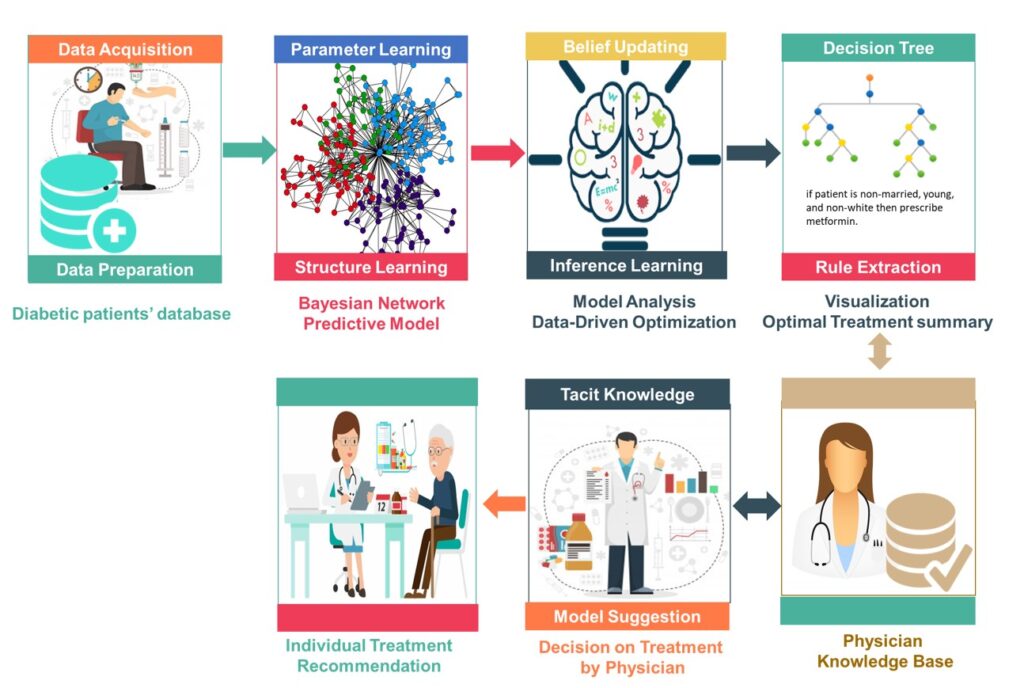
The number of people affected with diabetes in the world has been increasing over the past few decades. More than 463 million adults are living with diabetes. The figure is expected to rise to 552 million by 2030. The risks of death and cardiovascular events among diabetic patients are two to four times greater than those risks in the general population. Diabetes mellitus is associated with several comorbid conditions, such as hypertension, hyperlipidemia, and cardiovascular disorders. They are prescribed multiple drugs and Polypharmacy is an integral part of diabetic patients. So, in presence of comorbidity and polypharmacy diabetes management and treating T2D patients is so complex. This research aims to design a clinical decision analysis model to support the management of Type 2 diabetes (T2D) therapy.
We proposed a data-driven optimization approach to solve a sequential decision problem for diabetes management using multiple medications at hand. Using cross-sectional data collected from diabetic patients treated in the VA Medical Center in the US, we added optimization functionality in predictive analytics using Bayesian Network to characterize the optimal combination and sequence of diabetes medications considering patients’ discrepancies in chronic conditions and demographic characteristics to minimize the probability of 30-day mortality. We characterize conditions (based on the patient’s comorbidity and demographic information) under which each available treatment pathway should be prescribed for each patient group. It is done by considering both medications’ effectiveness and associated side effects (i.e., complications). On the decision analysis applications of Bayesian Networks, we utilized an optimization algorithm built on the notion of Markov blankets in Bayesian networks to characterize optimal treatment pathways in very complex Bayesian networks with several decision variables (antidiabetic medications) and state (comorbidities and demographic) variables. This algorithm identifies the smallest subset of variables sufficient to identify optimal decisions for patients’ treatment regimens. Any variables outside the identified subset will become conditionally independent of, hence irrelevant to, the target variable (i.e., mortality).
Experience of Disability Among Older Adults
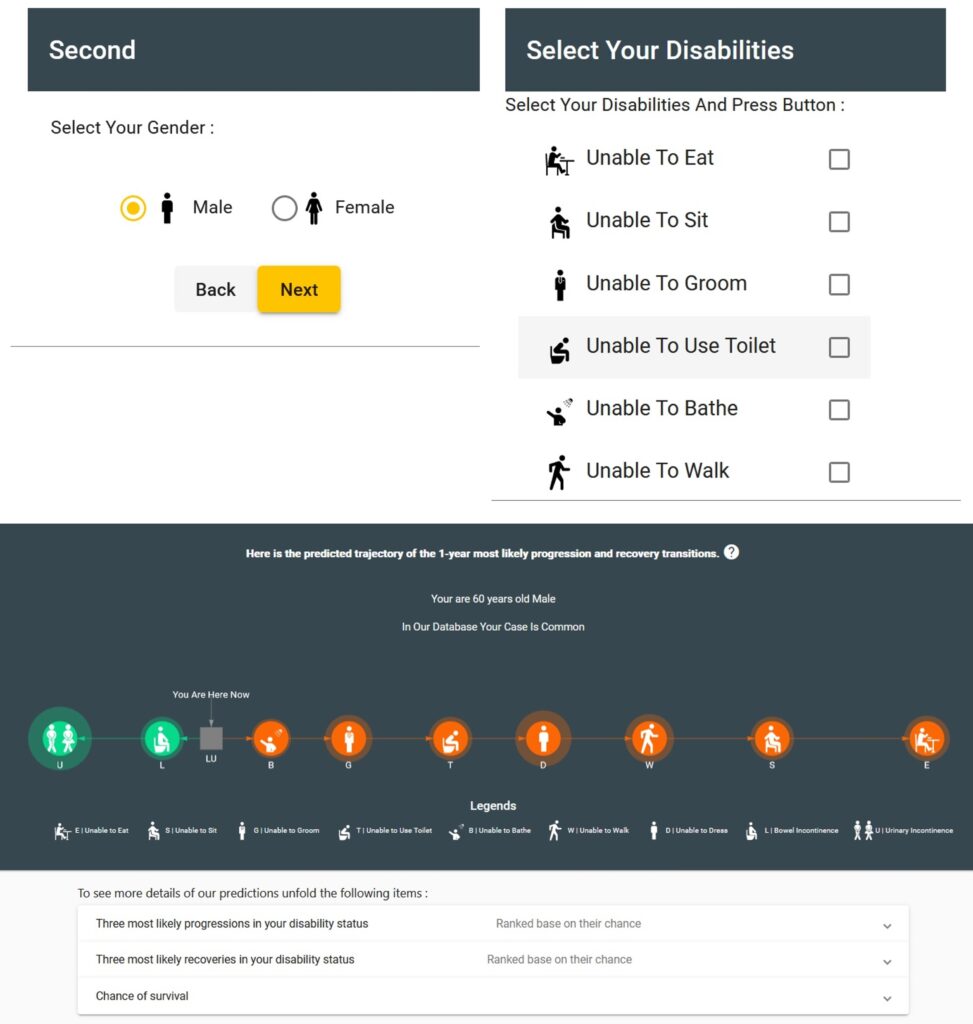
Little is known about how to guide patients, providers, families, and policymakers regarding expectations for the sequence of, likelihood, and time to functional loss and recovery of the activities of daily living (ADLs). Moreover, without benchmarks to gauge the time to improve or decline in functional abilities, it is difficult to assess the effectiveness of the intervention for recovery of functional loss. Leveraging machine learning algorithms and 1.8 million observations collected from 300,000 US Veteran Administrations Community Living Centers residents, we provide a data-driven analytical model of functional status change for residents in nursing homes.
In this research, we identify the most likely path(s) that elderlies follow from no disabilities to all nine categories of disabilities in the late stages of their lives. Particularly, we analyze the likelihood, the number of days until, and the sequence of functional decline, recovery, and death after each ADL. Establishing such a patient-centric benchmark is vital to ensuring optimal care for persons in nursing home settings. This information here can help in planning for end-of-life disabilities.
Addressing Alternate Level of Care Issues Facing Older Adults
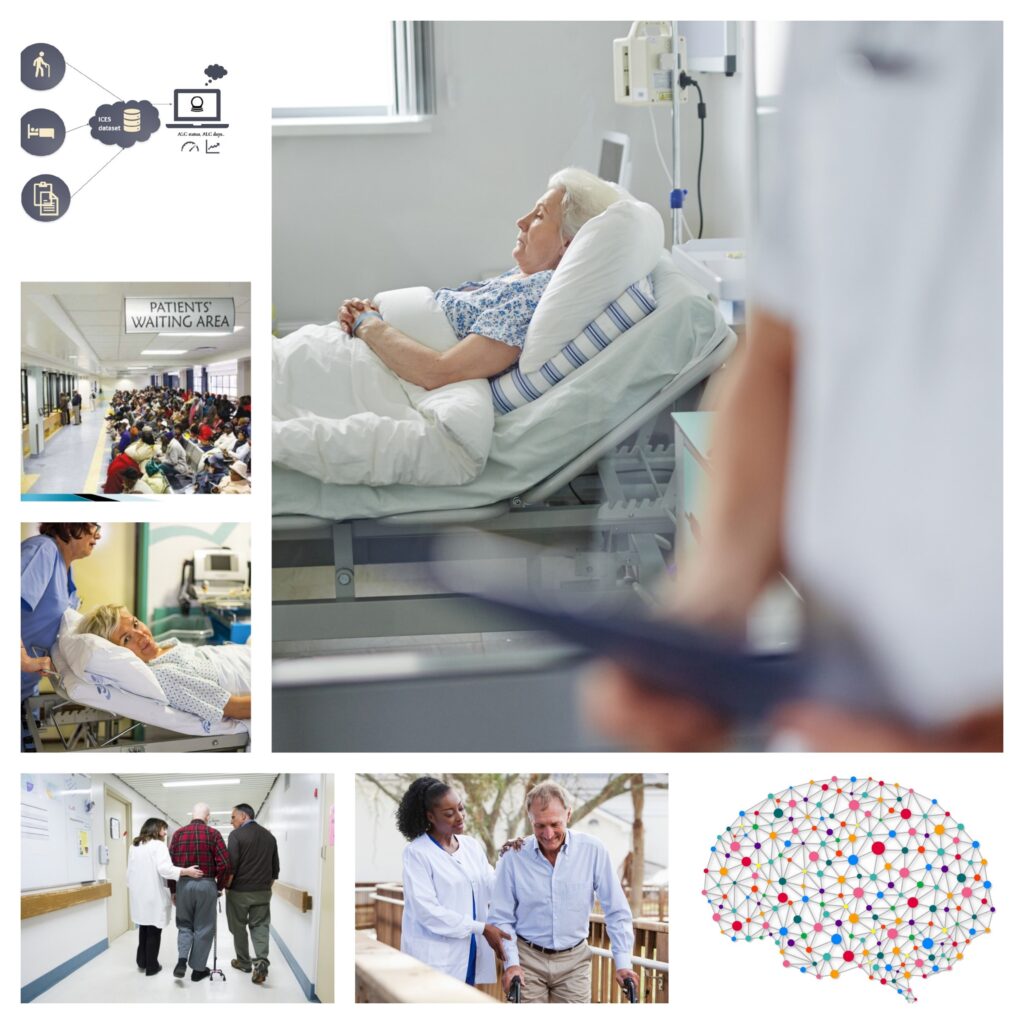
Delayed discharge, also known as Alternate Levels of Care (ALC) patients occupy an acute or post-acute bed despite no longer requiring the intensity of these resources while awaiting enrollment in community-based services (such as home care, rehabilitation, or long-term care). Whereas delayed discharge’s direct effects primarily relate to older patients, this issue also has a domino effect across the healthcare system, affecting all patient populations.
In the same context, I (and our team) have developed ML-based predictions to identify whether a patient would be designated as a delayed discharge patient from the emergency department (ED), the first patient contact point with the healthcare system, to the in-hospital stage. Early identification of such patients, beginning at admission to ED, could result in better resource management and decision-making by healthcare managers. The sooner decision-makers could be informed of patients with complex medical needs, such as delayed discharged patients, the better they could start planning their discharge from the hospital.
Clinicians and policymakers always emphasize the need for improving community-based care provision and service integration to minimize unwarranted hospital utilization. However, the limited availability of community-based services impedes the safe discharge of older adults, resulting in delayed discharge and inefficient use of acute care beds. Most of ALC patients are waiting for placements in alternative levels of care outside the hospital, such as Long-Term Care (LTC) facilities, which are often overcrowded and have limited capacity. One solution to this challenge is improving capacity planning and optimizing resource allocation in low-intensity care facilities. In a research project, in collaboration with an interdisciplinary team, we proposed a prediction-driven optimization model using large datasets from the Canadian older population to improve the utilization of the scarce LTC resources while optimizing the overall health of older adults awaiting placements in an LTC. In our model, the predicted future health state derived through ML is explicitly incorporated into the decision-making process to characterize optimal discharge planning supported by predictive information. We also develop and utilize a fully data-driven cost function/model personalized to the patient’s clinical characteristics and health status to estimate the hospital expenses incurred by the patient. This personalized cost function incorporates the immediate consequences of the decisions and their future implications for patient readmission.